The purpose of this study is to propose a new biomarker-based treatment outcome prediction model for improving local tumor control after radio-chemo therapy of cancer patients. The prediction model is developed based on belief function theory and sparsity learning to address the challenges of redundancy, heterogeneity, and uncertainty of radiomic and genomic features, relatively small-sized and unbalanced training samples for more accurate prediction. The model includes three stages of feature preparation, predictive feature subset selection, and outcome prediction. The model can select 1) most predictive feature subsets from relatively large amount of radiomic features extracted from pre- and/or in-treatment Positron emission tomography (PET) images, and 2) identify prognostic miRNA, HPV, clinical, and histopathologic biomarkers from given patient data. The selected features are combined with clinical and demographic features for treatment outcome prediction with an evidential k-nearest neighbor classifier. Performance and robustness of the proposed model was assessed with measures of feature selection stability, outcome prediction accuracy, and receiver operating characteristics analysis.
Methodology
The biomarker selection strategy is described in Figure 1. It is designed as the combination of our developed BFT-based method with the support from other machine-learning methods. The strategy will identify the most predictive biomarkers from the prepared features in each category (radiomics, miRNAs, HPV, clinical and histopathologic features).
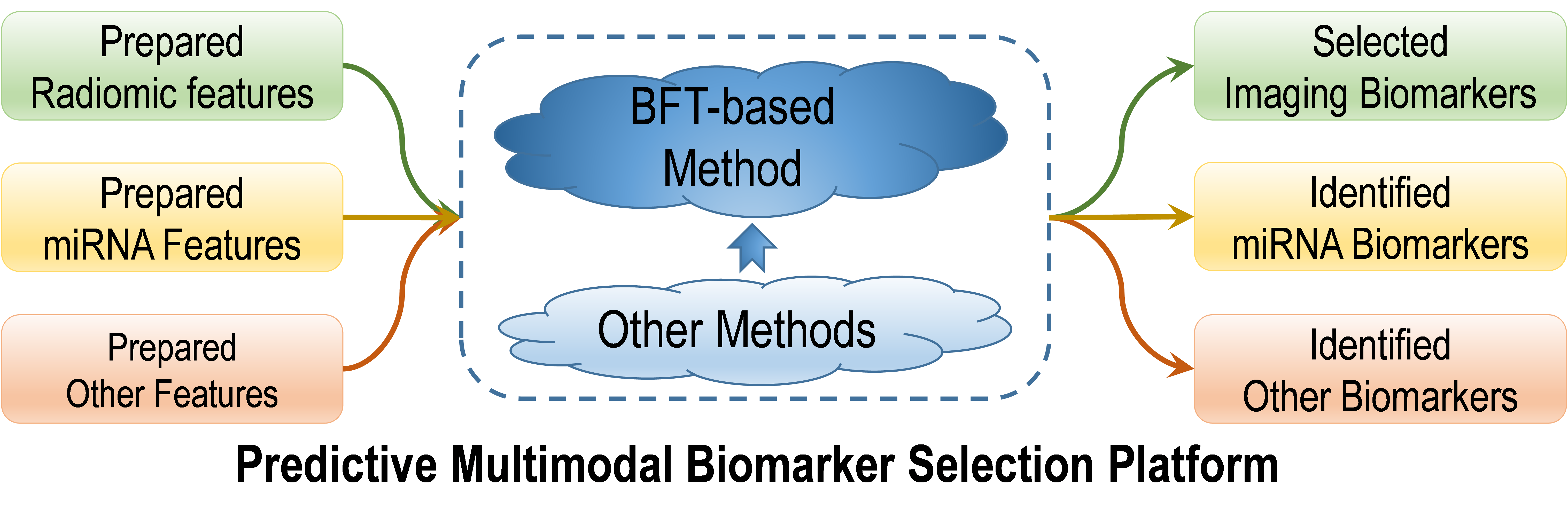
Results
To assess the prediction performance after feature selection, accuracy and AUC metrics were computed for the BFT-method and other eight methods. For all the compared methods except EFS and the model, the SVM was chosen as the default classifier; the EK-NN classifier was used with EFS and BFT. As shown in Figure 2, the proposed BFT-based method obtained better results than other methods on all four patient cohorts. In addition, the prediction accuracy and AUC corresponding to the model yielded the lowest values on the Esophagus patient cohort.
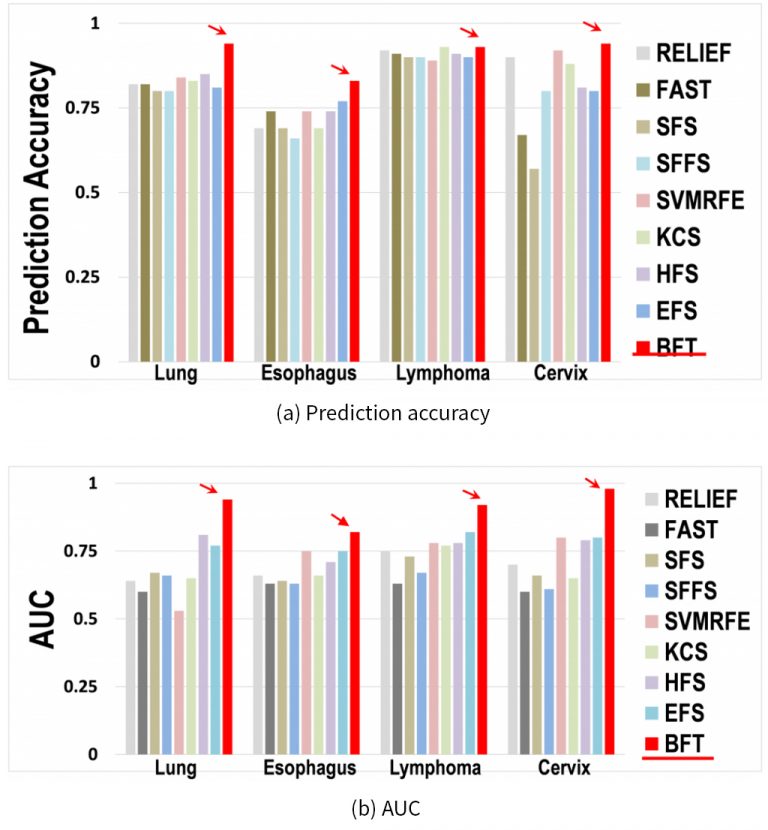
Related publications
1. Jian Wu, Chunfeng Lian, Su Ruan, Thomas Mazur, Sasa Mutic, Mark Anastasio, Perry Grigsby, Pierre Vera, Hua Li*, “Treatment Outcome Prediction for Cancer Patients based on Radiomics and Belief Function Theory”, IEEE Trans. on Radiation and Plasma Medical Sciences (RPMS), Special issue on Machine Learning in Radiation based Medical Sciences, 2019.
2. Jian Wu, Chunfeng Lian, Su Ruan, Sasa Mutic, Mark Anastasio, Hiram Gay, Wade Thorstad, Xiaowei Wang, Hua Li*. “Selecting Predictive Geometric Biomarkers for Oropharyngeal Cancer Treatment Prediction by Use of Advanced Machine Learning Method”, AAPM Annual Meeting, 2018. (Winner of The Jack Krohmer Junior Investigator Competition)
3. Ziang Zhu, Thomas Mazur, Chunfeng Lian, Su Ruan, Mark Anastasio, Jian Wu, Brian McClain, Jeffrey Williamson, Perry Grigsby, Sasa Mutic, Hua Li*, “Radiomics-Based Treatment Outcome Prediction Based on Belief Function Theory and Sparsity Learning”, AAPM Annual Meeting 2017.
4. Chunfeng Lian, Hua Li*, Thierry Denoeux, Hsin-Chen Chen, Clifford Robinson, Pierre Vera, Su Ruan, “Cancer Therapy Outcome Prediction Based On Dempster-Shafer Theory and PET Imaging”, AAPM Annual Meeting, 2015. (A finalist for the John R. Cameron Young Investigator competition)
5. Su Ruan, Hongmei Mi, Caroline Petitjean, Hua Li, Hsin-Chen Chen, Clifford Robinson, Bernard Dubray, Pierre Vera, “Robust Optimal Feature Selection for Lung Tumor Recurrence Prediction with PET Imaging”, ASTRO Annual Meeting, 2015.
6. Chunfeng Lian, Su Ruan, Thierry Denoeux, Hua Li, Pierre Vera, “Robust Cancer Treatment Outcome Prediction Dealing with Small-sized and Imbalanced Data from FED-PET Images”, 19th International Conference on Medical Image Computing and Computer Assisted Intervention (MICCAI’2016), Oct. 17-21, 2016, Athens, Greece, pp.61-69. Doi:10.1007/978-3-319-46723- 88
7. Chunfeng Lian, Su Ruan, Thierry Denoeux, Hua Li, Pierre Vera, “Dempster-Shafer Theory based Feature Selection with Sparse Constraint for Outcome Prediction in Cancer Therapy”, 18th International Conference on Medical Image Computing and Computer Assisted Intervention (MICCAI’2015), Oct. 5-9, 2015, Munich, Germany, LNCS 9351, PP: 695-702.