Deep learning (DL) techniques have been extensively utilized for medical image classification. Most DL-based classification networks are generally structured hierarchically and optimized through minimization of a single loss function measured at the end of the networks. However, such a single loss design could potentially lead to optimization of one specific value of interest but fail to leverage informative features from intermediate layers that might benefit classification performance and reduce the risk of overfitting. Recently, auxiliary convolutional neural networks (AuxCNNs) have been employed on top of traditional classification networks to facilitate the training of intermediate layers to improve classification performance and robustness. In this study, we proposed an adversarial learning-based AuxCNN to support the training of deep neural networks for medical image classification.
Methodology
The proposed framework is shown in Figure 1.. Two main innovations were adopted in our AuxCNN classification framework. First, the proposed AuxCNN architecture includes an image generator and an image discriminator for extracting more informative image features for medical image classification, motivated by the concept of generative adversarial network (GAN) and its impressive ability in approximating target data distribution. Second, a hybrid loss function is designed to guide the model training by incorporating different objectives of the classification network and AuxCNN to reduce overfitting.
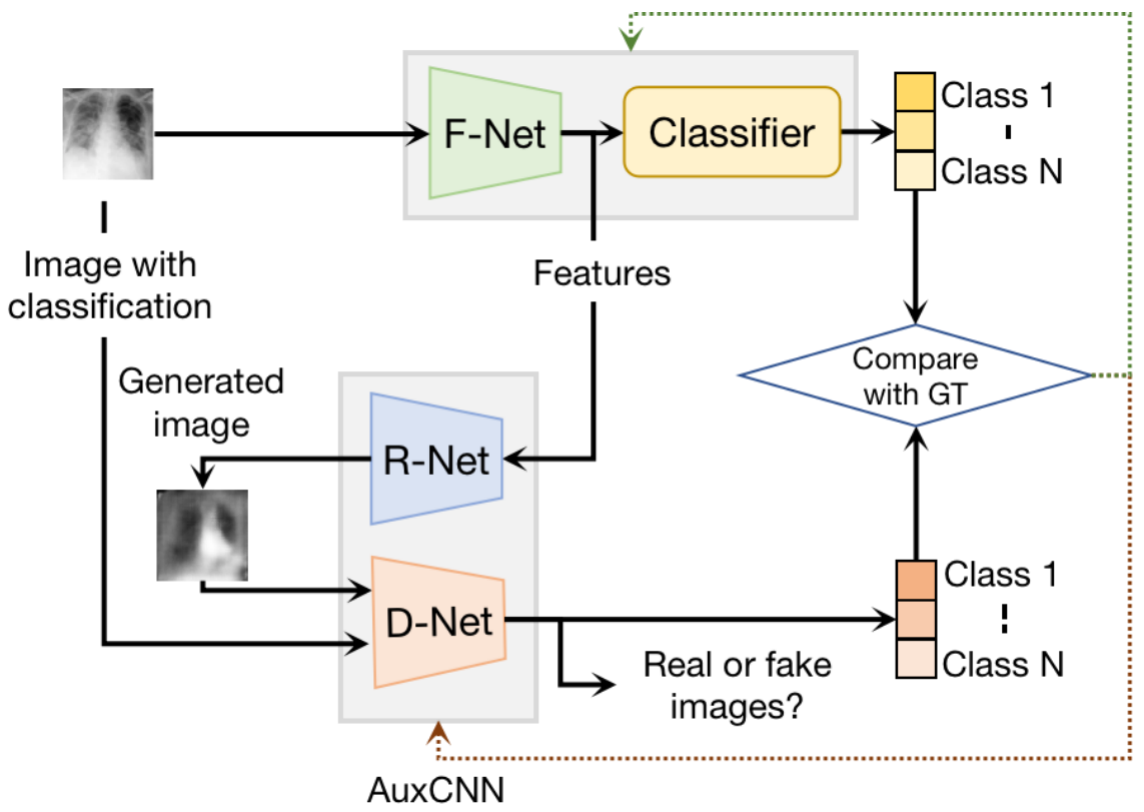
Results
The proposed framework was evaluated using two different datasets, a public COVID-19 patient dataset called COVIDx and an oropharyngeal squamous cell carcinoma (OPSCC) patient dataset. The experimental results demonstrated the superior classification performance of the proposed model. The effect of the network-related factors on classi cation performance was investigated. The classification performances obtained by different input image sizes and feature extraction network architectures are shown in the Figure 2.
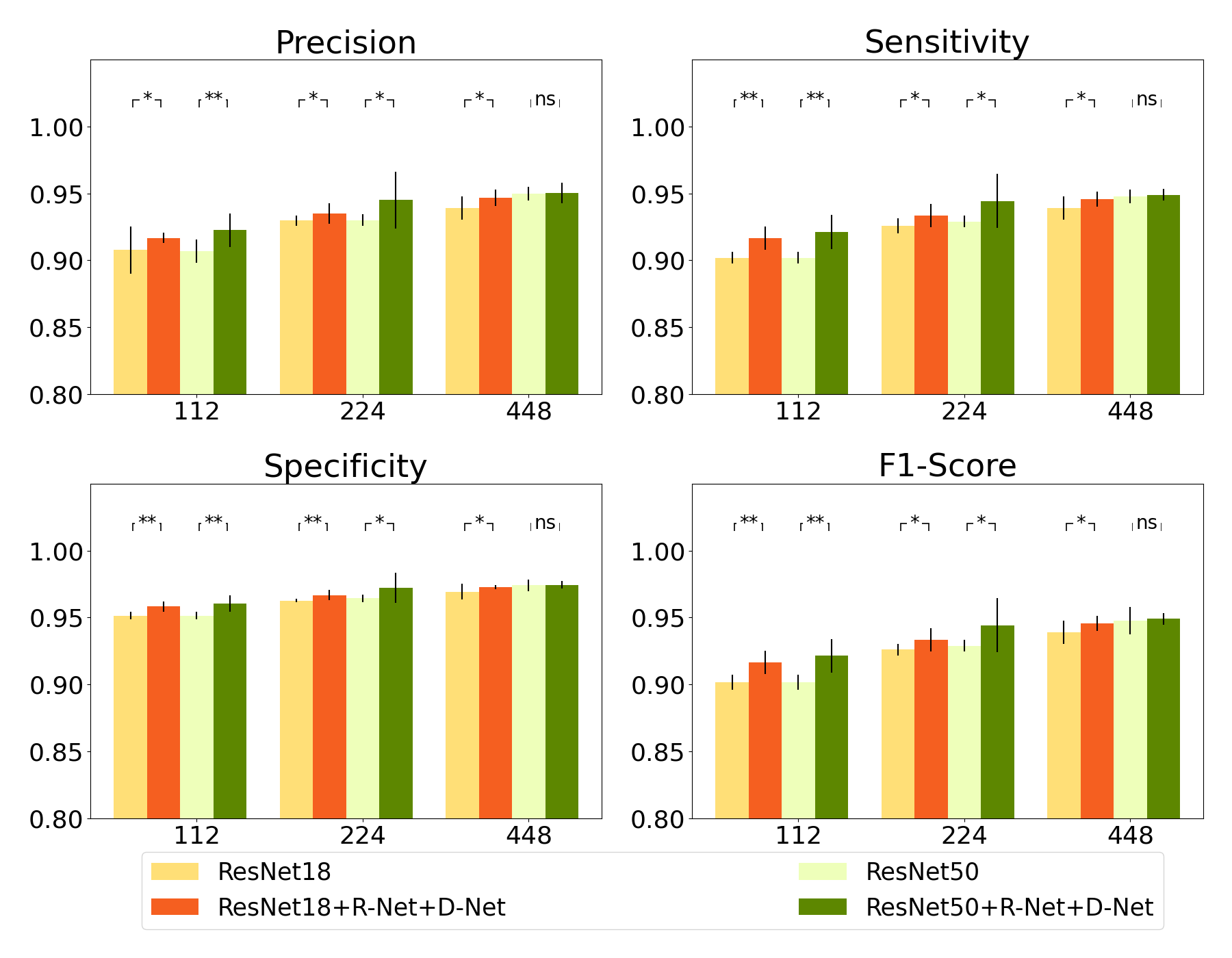
Related publications
1.Zong Fan, Ping Gong, Shanshan Tang, Christine U. Lee, Xiaohui Zhang, Shigao Chen, Pengfei Song, Hua Li*. Joint localization and classification of breast masses on ultrasound images using a novel auxiliary attention-based framework. In Proceedings.
2. Zong Fan, Shenghua He, Su Ruan, Xiaowei Wang, Hua Li, “Deep learning-based multi-class COVID-19 classification with x-ray images,” SPIE Medical Imaging Conference Proceedings, 2021, Oral presentation.
3. Zhimin Wang, Zong Fan, Lulu Sun, Xiaowei Wang, Hua Li, “Deep-supervised multiclass classification by use of digital histopathology images”, SPIE Medical Imaging Conference Proceedings, 2023